Monitor
forest tree health through the use of drones, satellites and data science
Identify and inspect
areas of potentially high fire risk
Monitor
ecosystems and assess biodiversity and wildlife populations
Evaluate
the capacity of drones to manage or prevent the expansion of infectious diseases affecting both feral and domestic animals.
Forestry and biodiversity monitoring
In UC4 the combination of different types of UAVs and cameras will be used to create optimised solutions for the specific scenarios: for tree health and fire risk monitoring - a multi-rotor drone, and for wildlife monitoring - a fixed-wing drone. The satellite imaging data will be used to detect possible tree stress, meanwhile multi-rotor drones will be used for detailed (high-resolution) monitoring of specific forest areas (including tree health and fire risks), while fixed-wing drones are becoming an efficient tool in forestry research and will be used for wildlife monitoring due to their capacity to cover vast areas and provide fast monitoring data. Since they can cover large areas of interest within a limited time frame, the operational costs of implementing UAVs are significantly reduced in comparison to other monitoring means, such as manned aerial surveys.
Technical Requirements
Pilot & Test Area
Area: Lithuania
55.36471734524808, 26.071057108020497
Lithuanian forest area based on
- prevailing tree species,
- the specific areas depending on environmental and other conditions.
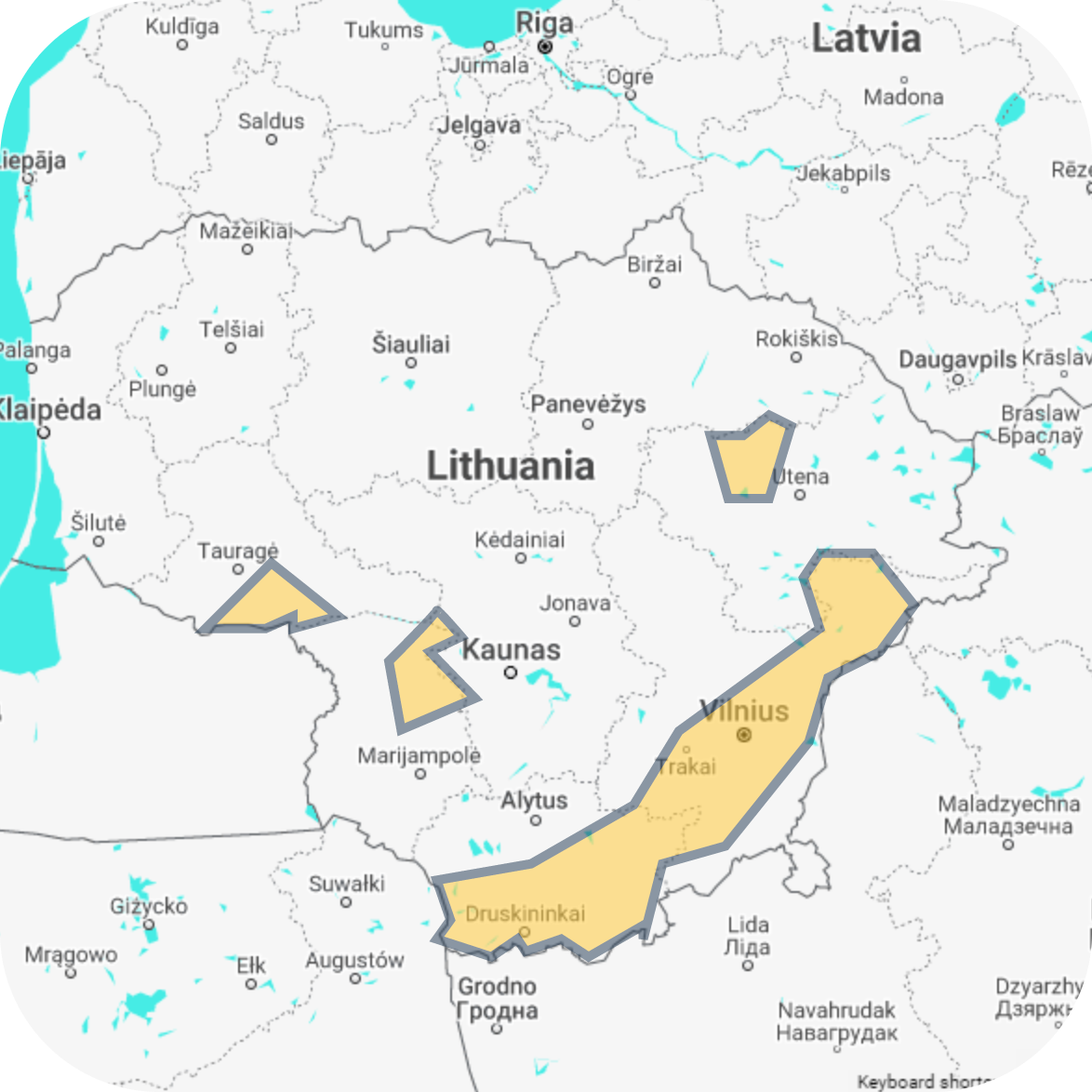
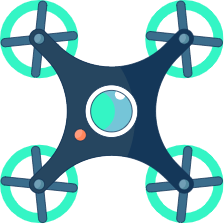
- DJI Matrice 600, DJI Phantom 4
- Aurelia X8 Standard (Octocopter)
- Volantex RC Ranger 2400 757-9 PNP
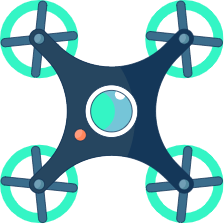
- RadioMaster TX16S + TBS V2 crossfire
- Specim Hyperspectral Camera
- Pixhawk 6C
- WIRIS Thermal imaging camera
- Gimbal stabilisation system
Methodology
Scenario 1 - Forest Tree Health
The methodology of the forest tree health monitoring will be based on the combined usage of the multispectral satellite imagery (Sentinel-2 MSI) and the UAV system consisting of the multi-rotor drone and VNIR-range hyperspectral camera. Forest tree health monitoring will consist of several steps of execution:
- The primary assessment of forest tree health from satellite images.
- Drone mission planning and execution over the satellite-identified unhealthy forest areas.
- Processing of hyperspectral drone sensing data.
- Detailed assessment of forest tree health symptoms using hyperspectral imaging data.
Scenario 2 - Wildfire Risk Monitoring
The data acquisition system for forest fire fuel mapping will consist of a multi-rotor drone, hyperspectral VNIR-range camera, and flight mission planning software. The use case scenario will be executed following the steps below:
- Drone mission planning over the forest test area.
- Drone flight execution and hyperspectral data collection over the forest area.
- Processing of UAV-collected hyperspectral data.
- Classification of forest fire fuel types in the hyperspectral image.
- Quantification and visualisation of forest fuel types and their availability in the forest test area.
Scenario 3 - Wild Boars Monitoring
The wild boars monitoring methodology is going to be developed as an improvement of the already piloted research and a prototype model in development by ART21 of an African swine fever control system that is based on remote aerial techniques and ML algorithms (TRL 5).
The developed prototype model and algorithms are presented in Figure 37 and their main characteristics are summarized in the following:
- Thermal data pre-processing and GPS data integration into thermal imagery and video data.
- Automatic wild boar detection in thermal images with AI models based on deep convolutional networks for object detection in images.
- Unique wild boar identification in different images and counting algorithms.